Artificial Intelligence and Machine Learning/Translation in Pharma Language Translation
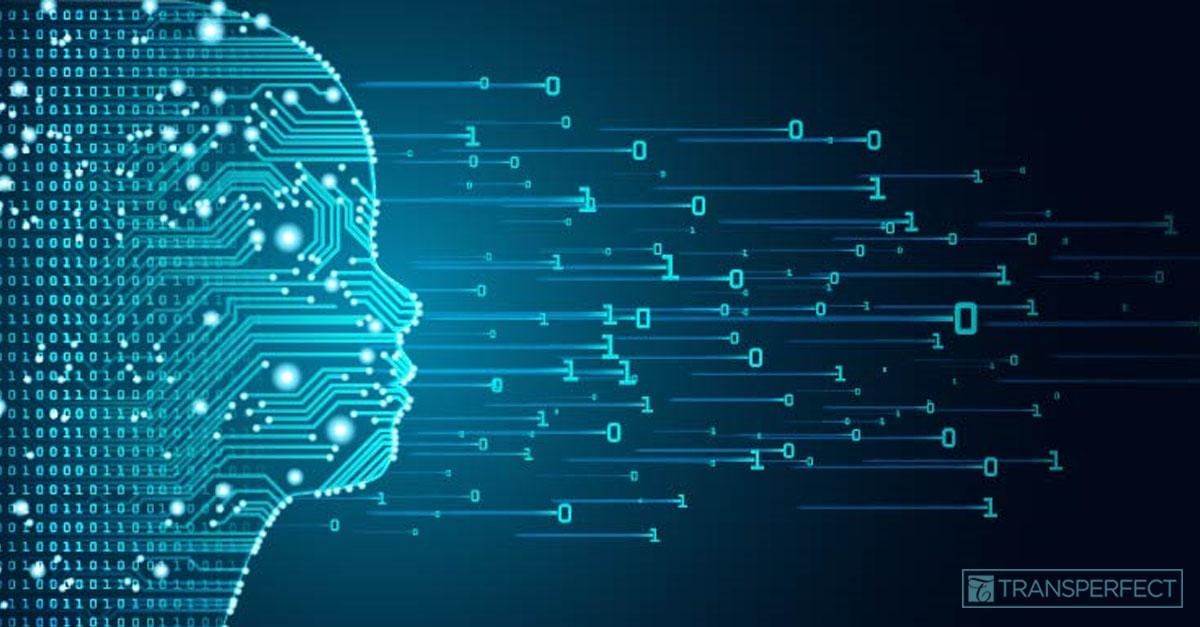
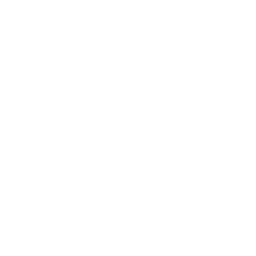
Artificial intelligence (AI) and machine learning (ML) in a nutshell: AI is a system that is tasked with the chance of success and not accuracy. ML is a system tasked with accuracy and not with success.
AI, in practice, has a lot of potential and also a lot of limitations. AI is being used to model, extrapolate, and automate tasks throughout the lifecycle of a drug (as it should be). The potential benefits are substantial, both in terms of clinical efficacy and financial savings. The low-hanging fruit was big data analysis for drug interactions, as the data is easily consumable and analyzed by today’s AI. Amassed medical data on drugs can be easily compared against patient medications to alert study teams to potential risks. Patient recruitment, monitoring, and retention by way of analyzing large EMR data sets, both structured and unstructured, is a point of focus and is a similarly accessible and important use case for AI. Pinpointing patient heterogeneity, securing patients who are more likely to match the ever-increasing inclusion/exclusion criteria and also have the capacity to respond to certain treatments.
AI, and in this case, neural language processing (NLP) could vastly speed up electronic phenotyping, which is a complex task of reviewing EMRs to find that suitable heterogeneous target patient population.
With all of the supposed power and promise of this advanced technology, what are the real, practical implications for clinical outcome assessments?
Machine Learning for "Reading" Clinical Content
Each day many of us interact with our personal assistant via speech and, for the most part, it a) recognizes us as long as our accent and intonation is clear and b) understands multiple languages. ML uses a wide variety of algometric techniques to understand what we are saying. NLP is one of those techniques that clinical researchers can leverage to identify patterns that can support drug development decisions, patient recruitment clusters, and drug interactions. NLP can be trained to “read” content such as scientific papers, EMRs, and physician notes. In processing and analyzing large sets of unstructured and structured data it can extrapolate outcomes. ML has already been used to analyze MRI scans to detect abnormalities earlier, thus saving lives. ML in clinical research can impact:
- Patient recruitment
- Drug visibility
- Drug interaction
- Identifying patients with similar biomarkers and health data to predict the chances of disease contraction/state
Machine Learning for Differential Diagnosis
The driving force behind ML enabling the processing of structured and unstructured data is deep neural learning, an algorithm with neuron nodes connected together like a web mirroring the human brain. Deep learning systems help to inform differential diagnosis. By using content-based image retrieval visible symptoms can be compared to a large set of data and increase diagnostic accuracy. It also addresses the capacity issues of a qualified workforce.
Machine Learning for Linguistic Relationships
ML can process structured and unstructured big data sets and extrapolate a predictable outcome. ML can process medical dictionaries (MedDRA, etc.) to augment its understanding of the relationship between the terms regardless of whether they are different contextually, semantically, or linguistically.
AI for Disease Response
AI can be applied in situations with a direct impact on the public health. AI technology will enable us to make predictions and anticipate the spread of contagious diseases, allowing for faster reactions and mobilization of the necessary support.
AI for Improved Outcomes
The access to all of this patient data improves patient outcomes. The amounts of available data are ever increasing, especially with new data collection technologies being developed, such as mirrors recording changes in your physical appearance and smart pills understanding your reactions to any form of medication.
These large data sets, when utilized correctly, can help pharmaceutical companies to understand the patient journey of their customers and to provide accurate insights into the efficacy of the products.
ML and AI clearly have benefits for much of the clinical study lifecycle, but how will they fair with clinical outcome assessments (COAs)?
- COAs depend on a systematic set of intertwined and related items/questions used in assessments
- Physiological and biological data can be gathered from site visits and physician follow-up examinations
COAs gather precisely how patients are experiencing the study and how they perceive the study drug performance.
Clinical Outcome Assessments and AI
Validated questionnaires are created specifically to capture the patient’s experiences in a study from a subjective point of view. Each of these instruments are scientifically proven and stress-tested to achieve a constant response each and every time. These instruments are sets of carefully chosen verbiage that allow study personnel to gain insight on how a study drug is performing and how it’s perceived by the subject.
Clearly, physiological and biometric data is captured during site visits and in physicians’ offices, but it’s this subjective feedback that could defeat AI, given the precise verbiage used in a validated instrument.
Independent words can be readily translated by machine translation (MT), but it’s the nuance, word selection, and the instrument in its totality that present the challenge. When translating a question there may be numerous permutations for a translation or translated word. The selection of these words and the subtle yet critical interpretation presents the conundrum. Moreover, the instrument is not merely one question after another, but a series of questions that act in concert with each other—a system of assessment items.
Human translators are selected to translate such assessments based on the familiarity and understanding of a) how the assessments are created and b) how a patient in the target disease state may respond to particular verbal stimuli.
The translation process for any validated assessment is well documented and is by far the most robust and complex methodology used in any clinical document translation. By virtue of how critical and subjective the assessments are, AI translation may not be at a point where it can take over from a human that specializes in said assessments.
Future Trials
Utilizing AI and ML will accelerate the development process in R&D and clinical trials. Being able to process data quicker will help to shorten the timelines for getting a drug on the market
Understanding an analysis of errors and wastage will have an impact on yields and waste during production processes. Outside of manufacturing it can be used to make any kind of process leaner and more efficient.
We are now gathering more data per patient in a trial than ever. We are not just capturing the screening data and the site visit data; we are capturing patient-reported outcome data, be it self-reported or clinician reported. We are also witnessing a growth in wearable sensors used to capture data from patients dynamically during a trial (pedometers, heart rate monitors, etc.). All of this big data, coupled with the ability to absorb structured and unstructured data sets, will have repercussions in how we carry out clinical trials today. The move to more targeted, shorter patient recruitment and drug interactions will directly impact the cost of executing a trial by today’s standards— a cost that has increased some 145% in the last 10 years.
Conclusion
AI and ML indeed have a place in clinical translation. They “translate” to COAs by virtue of the nature of the instruments; of the innate challenges in the systematic nature of a validated instrument (i.e., each item/question is conceptually and grammatically connected to each other). Used in unison, the measure delivers stable, consistent responses irrespective of the patient’s aging, remission, or regression status. That harmony of perfect translation is currently beyond the ability of MT and AI. AI is an evolving technology and can be constantly fine-tuned until it reaches a point of acceptable clinical accuracy tolerance. Perhaps in years to come it can capture the patient’s voice perfectly.
The value of a qualified, skilled, human outcome assessment translator has yet to be equaled…for now.